How to identify your customers' purchasing moment using MMM
- Nicolás Bonati
- Mar 31
- 2 min read
Updated: May 9
One of the greatest challenges in today's marketing is understanding which channels and actions truly influence the key conversion moment. Was it a social media ad? A Google search? A last-minute email? To answer these questions, many companies turn to multi-touch attribution (MTA) modeling, but it's also possible to capture this information using Marketing Mix Modeling (MMM), especially when combined with an appropriate channel modeling strategy.
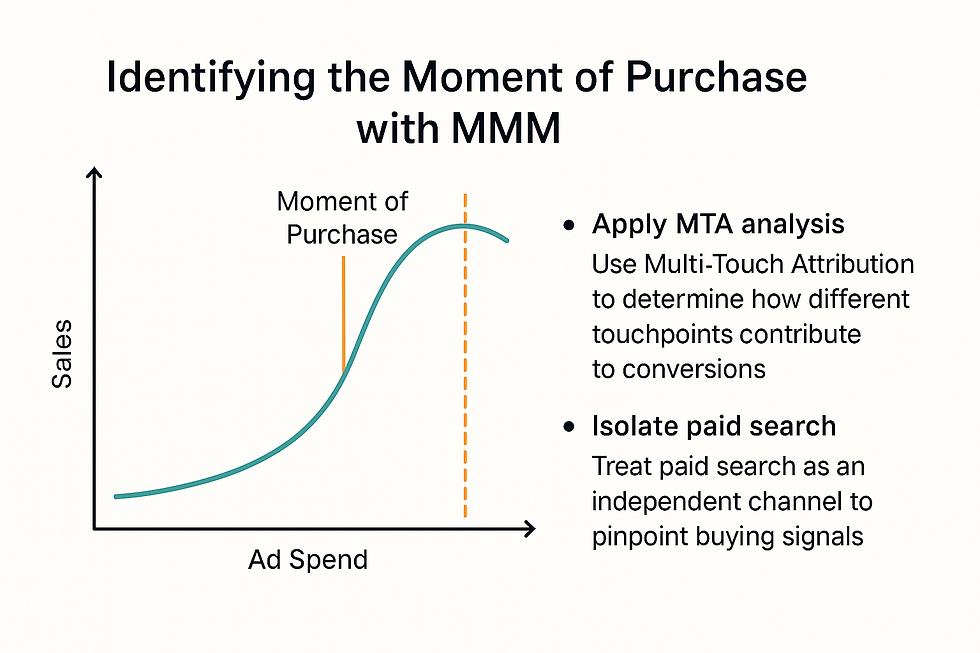
Can you identify the "moment of purchase" using MMM?
Yes. Although MMM traditionally works with aggregate data and evaluates macro-level effects, with a good data structure and channel segmentation, it's possible to get closer to the moment of purchase, even without individual data..
Strategies to capture the conversion point
1. Model closing touchpoints as independent channels. An effective way to capture the influence of the purchase moment is to separate channels where conversions typically occur (such as brand search, email marketing, or pricing pages) as independent variables in the model. This allows the model to directly estimate their contribution to total revenue, without diluting their effect among other media.
Real-world example: A dental clinic in Santiago used NeuroRadar to separate traffic from Google brand searches from other paid traffic. They found that over 30% of conversions came from brand searches made after viewing offline ads.
In the response curve: You'll notice that these channels have a more direct and efficient relationship between investment or volume and results. They tend to have curves with lower saturation, since they act as final triggers rather than demand generators.

2. Integrate MTA insights such as qualitative inputs or validations. MMM doesn't have access to user data, but can benefit from information derived from MTA models, such as the average time between first contact and conversion, or the frequency of certain conversion paths. This allows you to fine-tune the model or segment the data to properly assess the role of each channel.
Real-life example: A Chilean fintech combined its MMM model with attribution paths observed in Google Analytics. They found that 80% of converted users had previously interacted with educational content, so they included this channel as an additional explanatory variable in the MMM.
3. Enrich the model with direct intent variables. You can include signals that reflect high purchase intent (such as brand searches, direct traffic, or events like clicks on buy buttons) as explanatory variables within the model. These variables help capture the behavior of users close to converting, improving your understanding of the decision-making process.
Real-world example: A B2B software company added the number of pricing page views per week as a variable. The MMM model revealed that this variable had a strong correlation with sales closing, even surpassing clicks on Google Ads campaigns.
What can you get?
A more realistic estimate of the role of closing channels
A clear view of how much revenue is actually driven by performance media and which is just facilitating the last step
The possibility of optimizing your budget without overvaluing the final conversion channel
In summary
While MMM and MTA are different approaches, they are not mutually exclusive. Using MMM with a well-defined channel structure allows you to understand not only what generates demand, but also which channels are actively involved in the purchase moment. This allows you to build a more efficient, transparent, and profitable strategy.
Comments